|
Records |
Links |
|
Author |
Lobato, I.; Friedrich, T.; Van Aert, S. |


|
|
Title |
Deep convolutional neural networks to restore single-shot electron microscopy images |
Type |
A1 Journal Article |
|
Year  |
2024 |
Publication |
npj Computational Materials |
Abbreviated Journal |
npj Comput Mater |
|
|
Volume |
10 |
Issue |
1 |
Pages |
10 |
|
|
Keywords |
A1 Journal Article; Electron Microscopy for Materials Science (EMAT) ; |
|
|
Abstract |
Advanced electron microscopy techniques, including scanning electron microscopes (SEM), scanning transmission electron microscopes (STEM), and transmission electron microscopes (TEM), have revolutionized imaging capabilities. However, achieving high-quality experimental images remains a challenge due to various distortions stemming from the instrumentation and external factors. These distortions, introduced at different stages of imaging, hinder the extraction of reliable quantitative insights. In this paper, we will discuss the main sources of distortion in TEM and S(T)EM images, develop models to describe them, and propose a method to correct these distortions using a convolutional neural network. We validate the effectiveness of our method on a range of simulated and experimental images, demonstrating its ability to significantly enhance the signal-to-noise ratio. This improvement leads to a more reliable extraction of quantitative structural information from the images. In summary, our findings offer a robust framework to enhance the quality of electron microscopy images, which in turn supports progress in structural analysis and quantification in materials science and biology. |
|
|
Address |
|
|
|
Corporate Author |
|
Thesis |
|
|
|
Publisher |
|
Place of Publication |
|
Editor |
|
|
|
Language |
|
Wos |
001138183000001 |
Publication Date |
2024-01-09 |
|
|
Series Editor |
|
Series Title |
|
Abbreviated Series Title |
|
|
|
Series Volume |
|
Series Issue |
|
Edition |
|
|
|
ISSN |
2057-3960 |
ISBN |
|
Additional Links |
UA library record; WoS full record |
|
|
Impact Factor |
|
Times cited |
|
Open Access |
|
|
|
Notes |
This work was supported by the European Research Council (Grant 770887 PICOMETRICS to S.V.A.). The authors acknowledge financial support from the Research Foundation Flanders (FWO, Belgium) through project fundings (G034621N, G0A7723N and EOS 40007495). S.V.A. acknowledges funding from the University of Antwerp Research Fund (BOF). The authors thank Lukas Grünewald for data acquisition and support for Fig. 7. |
Approved |
Most recent IF: NA |
|
|
Call Number |
EMAT @ emat @c:irua:202714 |
Serial |
8994 |
|
Permanent link to this record |
|
|
|
|
Author |
Friedrich, T.; Yu, C.-P.; Verbeeck, J.; Van Aert, S. |
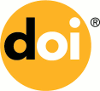
|
|
Title |
Phase object reconstruction for 4D-STEM using deep learning |
Type |
A1 Journal article |
|
Year  |
2023 |
Publication |
Microscopy and microanalysis |
Abbreviated Journal |
|
|
|
Volume |
29 |
Issue |
1 |
Pages |
395-407 |
|
|
Keywords |
A1 Journal article; Electron microscopy for materials research (EMAT) |
|
|
Abstract |
In this study, we explore the possibility to use deep learning for the reconstruction of phase images from 4D scanning transmission electron microscopy (4D-STEM) data. The process can be divided into two main steps. First, the complex electron wave function is recovered for a convergent beam electron diffraction pattern (CBED) using a convolutional neural network (CNN). Subsequently, a corresponding patch of the phase object is recovered using the phase object approximation. Repeating this for each scan position in a 4D-STEM dataset and combining the patches by complex summation yields the full-phase object. Each patch is recovered from a kernel of 3x3 adjacent CBEDs only, which eliminates common, large memory requirements and enables live processing during an experiment. The machine learning pipeline, data generation, and the reconstruction algorithm are presented. We demonstrate that the CNN can retrieve phase information beyond the aperture angle, enabling super-resolution imaging. The image contrast formation is evaluated showing a dependence on the thickness and atomic column type. Columns containing light and heavy elements can be imaged simultaneously and are distinguishable. The combination of super-resolution, good noise robustness, and intuitive image contrast characteristics makes the approach unique among live imaging methods in 4D-STEM. |
|
|
Address |
|
|
|
Corporate Author |
|
Thesis |
|
|
|
Publisher |
|
Place of Publication |
|
Editor |
|
|
|
Language |
|
Wos |
001033590800038 |
Publication Date |
2023-01-12 |
|
|
Series Editor |
|
Series Title |
|
Abbreviated Series Title |
|
|
|
Series Volume |
|
Series Issue |
|
Edition |
|
|
|
ISSN |
1431-9276 |
ISBN |
|
Additional Links |
UA library record; WoS full record; WoS citing articles |
|
|
Impact Factor |
2.8 |
Times cited |
1 |
Open Access |
OpenAccess |
|
|
Notes |
We acknowledge funding from the European Research Council (ERC) under the European Union's Horizon 2020 research and innovation program (grant agreement no. 770887 PICOMETRICS) and funding from the European Union's Horizon 2020 research and innovation program under grant agreement No. 823717 ESTEEM3. J.V. and S.V.A acknowledge funding from the University of Antwerp through a TOP BOF project. The direct electron detector (Merlin, Medipix3, Quantum Detectors) was funded by the Hercules fund from the Flemish Government. This work was supported by the FWO and FNRS within the 2Dto3D project of the EOS program (grant number 30489208). |
Approved |
Most recent IF: 2.8; 2023 IF: 1.891 |
|
|
Call Number |
UA @ admin @ c:irua:198221 |
Serial |
8912 |
|
Permanent link to this record |
|
|
|
|
Author |
Dinger, J.; Friedrich, T.; Reimann, T.; Toepfer, J. |
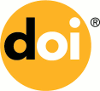
|
|
Title |
NiMn₂O₄ revisited : temperature-dependent cation distribution from in situ neutron diffraction and thermopower studies |
Type |
A1 Journal article |
|
Year  |
2023 |
Publication |
Journal of the American Ceramic Society |
Abbreviated Journal |
|
|
|
Volume |
106 |
Issue |
3 |
Pages |
1834-1847 |
|
|
Keywords |
A1 Journal article; Electron microscopy for materials research (EMAT) |
|
|
Abstract |
The cation distribution of the negative temperature coefficient (NTC) thermistor spinel NiMn2O4 was studied in the temperature range from 55 to 900 degrees C, using a combined in situ neutron diffraction and thermopower study. Rietveld refinements of in situ neutron diffraction data reveal a temperature dependence of the degree of inversion with an inversion parameter of 0.70(1) at 900 degrees C and 0.87(1) at 55 degrees C. Thermopower measurements were evaluated using a modified Heikes formula, and the [Mn4+]/[Mn3+] ratio on octahedral sites of the spinel structure was calculated considering spin and orbital degeneracy. The inversion degree and disproportionation parameter, determined independently as function of temperature, were used to calculate the cation distribution of NiMn2O4 in the whole temperature range. At high temperature, within the stability range of the spinel, the cation distribution is characterized by a moderate degree of inversion with a concentration of NiB2+${\mathrm{Ni}}\mathrm{B}< |
|
|
Address |
|
|
|
Corporate Author |
|
Thesis |
|
|
|
Publisher |
|
Place of Publication |
|
Editor |
|
|
|
Language |
|
Wos |
000880360600001 |
Publication Date |
2022-10-21 |
|
|
Series Editor |
|
Series Title |
|
Abbreviated Series Title |
|
|
|
Series Volume |
|
Series Issue |
|
Edition |
|
|
|
ISSN |
0002-7820 |
ISBN |
|
Additional Links |
UA library record; WoS full record |
|
|
Impact Factor |
3.9 |
Times cited |
|
Open Access |
OpenAccess |
|
|
Notes |
|
Approved |
Most recent IF: 3.9; 2023 IF: 2.841 |
|
|
Call Number |
UA @ admin @ c:irua:192087 |
Serial |
7326 |
|
Permanent link to this record |
|
|
|
|
Author |
Friedrich, T. |

|
|
Title |
Quantifying atomic structures using neural networks from 4D scanning transmission electron microscopy (STEM) datasets |
Type |
Doctoral thesis |
|
Year  |
2023 |
Publication |
|
Abbreviated Journal |
|
|
|
Volume |
|
Issue |
|
Pages |
127 p. |
|
|
Keywords |
Doctoral thesis; Electron microscopy for materials research (EMAT) |
|
|
Abstract |
Nanoscience and nanotechnologies are of immense importance across many fields of science and for numerous practical applications. In this context, scanning transmission electron microscopy (STEM) and 4D-STEM are among the most powerful characterization methods at the atomic scale. Annular dark-field (ADF)-STEM can be used to quantify atomic structures in 3D by counting atoms based on a single projection image. In 4D-STEM a full diffraction pattern is recorded at each scan step, which enables more dose efficient imaging and the utilization of various advanced imaging modalities, which can however be complex and slow. Both, STEM and 4D-STEM suffer from noise and distortions. In the first section of this work the most important of these distortions are discussed and it is shown how image restoration with a dedicated convolutional neural network (CNN) can be beneficial for atomic structure quantifications in ADF-STEM. In the second part, a new 4D-STEM imaging method real-time-integrated-centre-of-mass (riCOM) is introduced, which is a very dose-efficient and fast algorithm that enables unprecedented live-imaging capabilities for 4D-STEM. It is based on the integrated centre-of-mass approach, but is reformulated with variable integration ranges and optional filters, which allows for a tunable contrast transfer function. This enables the imaging of light and heavy elements simultaneously at very low doses. In the third part another new 4D-STEM method, coined AIRPI (AI-assisted rapid phase imaging) is introduced, which uses a CNN to retrieve a patch of the specimen's phase image for each scan position, based on the diffraction patterns in the probe's immediate surroundings. This allows also live imaging in principle and surpasses comparable state-of-the-art algorithms in terms of resolution also at low doses. Different atomic columns can be reliably distinguished over a wide range of atomic numbers, enabling a very good image interpretability. Further, AIRPI can recover low frequency image components, which preserves thickness information. This is a unique and important feature which could make quantitative 4D-STEM feasible. |
|
|
Address |
|
|
|
Corporate Author |
|
Thesis |
|
|
|
Publisher |
|
Place of Publication |
|
Editor |
|
|
|
Language |
|
Wos |
|
Publication Date |
|
|
|
Series Editor |
|
Series Title |
|
Abbreviated Series Title |
|
|
|
Series Volume |
|
Series Issue |
|
Edition |
|
|
|
ISSN |
|
ISBN |
|
Additional Links |
UA library record |
|
|
Impact Factor |
|
Times cited |
|
Open Access |
|
|
|
Notes |
|
Approved |
Most recent IF: NA |
|
|
Call Number |
UA @ admin @ c:irua:196826 |
Serial |
8919 |
|
Permanent link to this record |
|
|
|
|
Author |
Yu, C.-P.; Friedrich, T.; Jannis, D.; Van Aert, S.; Verbeeck, J. |


|
|
Title |
Real-Time Integration Center of Mass (riCOM) Reconstruction for 4D STEM |
Type |
A1 Journal article |
|
Year  |
2022 |
Publication |
Microscopy and microanalysis |
Abbreviated Journal |
Microsc Microanal |
|
|
Volume |
|
Issue |
|
Pages |
1-12 |
|
|
Keywords |
A1 Journal article; Engineering sciences. Technology; Electron microscopy for materials research (EMAT) |
|
|
Abstract |
A real-time image reconstruction method for scanning transmission electron microscopy (STEM) is proposed. With an algorithm requiring only the center of mass of the diffraction pattern at one probe position at a time, it is able to update the resulting image each time a new probe position is visited without storing any intermediate diffraction patterns. The results show clear features at high spatial frequency, such as atomic column positions. It is also demonstrated that some common post-processing methods, such as band-pass filtering, can be directly integrated in the real-time processing flow. Compared with other reconstruction methods, the proposed method produces high-quality reconstructions with good noise robustness at extremely low memory and computational requirements. An efficient, interactive open source implementation of the concept is further presented, which is compatible with frame-based, as well as event-based camera/file types. This method provides the attractive feature of immediate feedback that microscope operators have become used to, for example, conventional high-angle annular dark field STEM imaging, allowing for rapid decision-making and fine-tuning to obtain the best possible images for beam-sensitive samples at the lowest possible dose. |
|
|
Address |
|
|
|
Corporate Author |
|
Thesis |
|
|
|
Publisher |
|
Place of Publication |
|
Editor |
|
|
|
Language |
|
Wos |
000792176100001 |
Publication Date |
2022-04-25 |
|
|
Series Editor |
|
Series Title |
|
Abbreviated Series Title |
|
|
|
Series Volume |
|
Series Issue |
|
Edition |
|
|
|
ISSN |
1431-9276 |
ISBN |
|
Additional Links |
UA library record; WoS full record; WoS citing articles |
|
|
Impact Factor |
2.8 |
Times cited |
7 |
Open Access |
OpenAccess |
|
|
Notes |
Bijzonder Onderzoeksfonds UGent; H2020 European Research Council, 770887 ; H2020 European Research Council, 823717 ; H2020 European Research Council, ESTEEM3 / 823717 ; H2020 European Research Council, PICOMETRICS / 770887 ; Fonds Wetenschappelijk Onderzoek, 30489208 ; Herculesstichting; esteem3reported; esteem3jra |
Approved |
Most recent IF: 2.8 |
|
|
Call Number |
EMAT @ emat @c:irua:188538 |
Serial |
7068 |
|
Permanent link to this record |
|
|
|
|
Author |
Sentürk, D.G.; De Backer, A.; Friedrich, T.; Van Aert, S. |


|
|
Title |
Optimal experiment design for element specific atom counting using multiple annular dark field scanning transmission electron microscopy detectors |
Type |
A1 Journal article |
|
Year  |
2022 |
Publication |
Ultramicroscopy |
Abbreviated Journal |
Ultramicroscopy |
|
|
Volume |
242 |
Issue |
|
Pages |
113626 |
|
|
Keywords |
A1 Journal article; Electron microscopy for materials research (EMAT) |
|
|
Abstract |
This paper investigates the possible benefits for counting atoms of different chemical nature when analysing multiple 2D scanning transmission electron microscopy (STEM) images resulting from independent annular dark field (ADF) detector regimes. To reach this goal, the principles of statistical detection theory are used to quantify the probability of error when determining the number of atoms in atomic columns consisting of multiple types of elements. In order to apply this theory, atom-counting is formulated as a statistical hypothesis test, where each hypothesis corresponds to a specific number of atoms of each atom type in an atomic column. The probability of error, which is limited by the unavoidable presence of electron counting noise, can then be computed from scattering-cross sections extracted from multiple ADF STEM images. Minimisation of the probability of error as a function of the inner and outer angles of a specified number of independent ADF collection regimes results in optimal experimental designs. Based on simulations of spherical Au@Ag and Au@Pt core–shell nanoparticles, we investigate how the combination of two non-overlapping detector regimes helps to improve the probability of error when unscrambling two types of atoms. In particular, the combination of a narrow low angle ADF detector with a detector formed by the remaining annular collection regime is found to be optimal. The benefit is more significant if the atomic number Z difference becomes larger. In
addition, we show the benefit of subdividing the detector regime into three collection areas for heterogeneous nanostructures based on a structure consisting of three types of elements, e.g., a mixture of Au, Ag and Al atoms. Finally, these results are compared with the probability of error resulting when one would ultimately use a pixelated 4D STEM detector and how this could help to further reduce the incident electron dose. |
|
|
Address |
|
|
|
Corporate Author |
|
Thesis |
|
|
|
Publisher |
|
Place of Publication |
|
Editor |
|
|
|
Language |
|
Wos |
000873778100001 |
Publication Date |
0000-00-00 |
|
|
Series Editor |
|
Series Title |
|
Abbreviated Series Title |
|
|
|
Series Volume |
|
Series Issue |
|
Edition |
|
|
|
ISSN |
0304-3991 |
ISBN |
|
Additional Links |
UA library record; WoS full record; WoS citing articles |
|
|
Impact Factor |
2.2 |
Times cited |
|
Open Access |
OpenAccess |
|
|
Notes |
This work was supported by the European Research Council (Grant 770887 PICOMETRICS to S. Van Aert and Grant 823717 ESTEEM3). The authors acknowledge financial support from the Research Foundation Flanders (FWO, Belgium) through project fundings (G.0346.21N and EOS 30489208) and a postdoctoral grant to A. De Backer. S. Van Aert acknowledges funding from the University of Antwerp Research fund (BOF).; esteem3reported; esteem3jra |
Approved |
Most recent IF: 2.2 |
|
|
Call Number |
EMAT @ emat @c:irua:190925 |
Serial |
7118 |
|
Permanent link to this record |
|
|
|
|
Author |
Friedrich, T.; Yu, C.-P.; Verbeek, J.; Pennycook, T.; Van Aert, S. |


|
|
Title |
Phase retrieval from 4-dimensional electron diffraction datasets |
Type |
P1 Proceeding |
|
Year  |
2021 |
Publication |
Proceedings
T2 – IEEE International Conference on Image Processing (ICIP), SEP 19-22, 2021, Electr. network |
Abbreviated Journal |
|
|
|
Volume |
|
Issue |
|
Pages |
3453-3457 |
|
|
Keywords |
P1 Proceeding; Engineering sciences. Technology; Electron microscopy for materials research (EMAT) |
|
|
Abstract |
We present a computational imaging mode for large scale electron microscopy data, which retrieves a complex wave from noisy/sparse intensity recordings using a deep learning approach and subsequently reconstructs an image of the specimen from the Convolutional Neural Network (CNN) predicted exit waves. We demonstrate that an appropriate forward model in combination with open data frameworks can be used to generate large synthetic datasets for training. In combination with augmenting the data with Poisson noise corresponding to varying dose-values, we effectively eliminate overfitting issues. The U-NET[1] based architecture of the CNN is adapted to the task at hand and performs well while maintaining a relatively small size and fast performance. The validity of the approach is confirmed by comparing the reconstruction to well-established methods using simulated, as well as real electron microscopy data. The proposed method is shown to be effective particularly in the low dose range, evident by strong suppression of noise, good spatial resolution, and sensitivity to different atom types, enabling the simultaneous visualisation of light and heavy elements and making different atomic species distinguishable. Since the method acts on a very local scale and is comparatively fast it bears the potential to be used for near-real-time reconstruction during data acquisition. |
|
|
Address |
|
|
|
Corporate Author |
|
Thesis |
|
|
|
Publisher |
|
Place of Publication |
|
Editor |
|
|
|
Language |
|
Wos |
000819455103114 |
Publication Date |
2021-08-23 |
|
|
Series Editor |
|
Series Title |
|
Abbreviated Series Title |
|
|
|
Series Volume |
|
Series Issue |
|
Edition |
|
|
|
ISSN |
978-1-6654-4115-5 |
ISBN |
|
Additional Links |
UA library record; WoS full record; WoS citing articles |
|
|
Impact Factor |
|
Times cited |
|
Open Access |
OpenAccess |
|
|
Notes |
|
Approved |
Most recent IF: NA |
|
|
Call Number |
UA @ admin @ c:irua:189462 |
Serial |
7089 |
|
Permanent link to this record |