|
Record |
Links |
|
Author |
Friedrich, T.; Yu, C.-P.; Verbeeck, J.; Van Aert, S. |
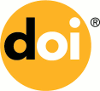
|
|
Title |
Phase object reconstruction for 4D-STEM using deep learning |
Type |
A1 Journal article |
|
Year  |
2023 |
Publication |
Microscopy and microanalysis |
Abbreviated Journal |
|
|
|
Volume |
29 |
Issue |
1 |
Pages |
395-407 |
|
|
Keywords |
A1 Journal article; Electron microscopy for materials research (EMAT) |
|
|
Abstract |
In this study, we explore the possibility to use deep learning for the reconstruction of phase images from 4D scanning transmission electron microscopy (4D-STEM) data. The process can be divided into two main steps. First, the complex electron wave function is recovered for a convergent beam electron diffraction pattern (CBED) using a convolutional neural network (CNN). Subsequently, a corresponding patch of the phase object is recovered using the phase object approximation. Repeating this for each scan position in a 4D-STEM dataset and combining the patches by complex summation yields the full-phase object. Each patch is recovered from a kernel of 3x3 adjacent CBEDs only, which eliminates common, large memory requirements and enables live processing during an experiment. The machine learning pipeline, data generation, and the reconstruction algorithm are presented. We demonstrate that the CNN can retrieve phase information beyond the aperture angle, enabling super-resolution imaging. The image contrast formation is evaluated showing a dependence on the thickness and atomic column type. Columns containing light and heavy elements can be imaged simultaneously and are distinguishable. The combination of super-resolution, good noise robustness, and intuitive image contrast characteristics makes the approach unique among live imaging methods in 4D-STEM. |
|
|
Address |
|
|
|
Corporate Author |
|
Thesis |
|
|
|
Publisher |
|
Place of Publication |
|
Editor |
|
|
|
Language |
|
Wos |
001033590800038 |
Publication Date |
2023-01-12 |
|
|
Series Editor |
|
Series Title |
|
Abbreviated Series Title |
|
|
|
Series Volume |
|
Series Issue |
|
Edition |
|
|
|
ISSN |
1431-9276 |
ISBN |
|
Additional Links |
UA library record; WoS full record; WoS citing articles |
|
|
Impact Factor |
2.8 |
Times cited |
1 |
Open Access |
OpenAccess |
|
|
Notes |
We acknowledge funding from the European Research Council (ERC) under the European Union's Horizon 2020 research and innovation program (grant agreement no. 770887 PICOMETRICS) and funding from the European Union's Horizon 2020 research and innovation program under grant agreement No. 823717 ESTEEM3. J.V. and S.V.A acknowledge funding from the University of Antwerp through a TOP BOF project. The direct electron detector (Merlin, Medipix3, Quantum Detectors) was funded by the Hercules fund from the Flemish Government. This work was supported by the FWO and FNRS within the 2Dto3D project of the EOS program (grant number 30489208). |
Approved |
Most recent IF: 2.8; 2023 IF: 1.891 |
|
|
Call Number |
UA @ admin @ c:irua:198221 |
Serial |
8912 |
|
Permanent link to this record |