|
Records |
Links |
|
Author |
Soltan, S.; Macke, S.; Ilse, S.E.; Pennycook, T.; Zhang, Z.L.; Christiani, G.; Benckiser, E.; Schuetz, G.; Goering, E. |
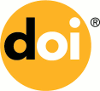
|
|
Title |
Ferromagnetic order controlled by the magnetic interface of LaNiO3/La2/3Ca1/3MnO3 superlattices |
Type |
A1 Journal article |
|
Year |
2023 |
Publication |
Scientific reports |
Abbreviated Journal |
|
|
|
Volume |
13 |
Issue |
1 |
Pages |
1-9 |
|
|
Keywords |
A1 Journal article; Engineering sciences. Technology; Electron microscopy for materials research (EMAT) |
|
|
Abstract |
Interface engineering in complex oxide superlattices is a growing field, enabling manipulation of the exceptional properties of these materials, and also providing access to new phases and emergent physical phenomena. Here we demonstrate how interfacial interactions can induce a complex charge and spin structure in a bulk paramagnetic material. We investigate a superlattice (SLs) consisting of paramagnetic LaNiO3 (LNO) and highly spin-polarized ferromagnetic La2/3Ca1/3MnO3 (LCMO), grown on SrTiO3 (001) substrate. We observed emerging magnetism in LNO through an exchange bias mechanism at the interfaces in X-ray resonant magnetic reflectivity. We find non-symmetric interface induced magnetization profiles in LNO and LCMO which we relate to a periodic complex charge and spin superstructure. High resolution scanning transmission electron microscopy images reveal that the upper and lower interfaces exhibit no significant structural variations. The different long range magnetic order emerging in LNO layers demonstrates the enormous potential of interfacial reconstruction as a tool for tailored electronic properties. |
|
|
Address |
|
|
|
Corporate Author |
|
Thesis |
|
|
|
Publisher |
|
Place of Publication |
|
Editor |
|
|
|
Language |
|
Wos  |
000985158100013 |
Publication Date |
2023-03-26 |
|
|
Series Editor |
|
Series Title |
|
Abbreviated Series Title |
|
|
|
Series Volume |
|
Series Issue |
|
Edition |
|
|
|
ISSN |
2045-2322 |
ISBN |
|
Additional Links |
UA library record; WoS full record; WoS citing articles |
|
|
Impact Factor |
4.6 |
Times cited |
|
Open Access |
OpenAccess |
|
|
Notes |
|
Approved |
Most recent IF: 4.6; 2023 IF: 4.259 |
|
|
Call Number |
UA @ admin @ c:irua:197426 |
Serial |
8867 |
|
Permanent link to this record |
|
|
|
|
Author |
Annys, A.; Jannis, D.; Verbeeck, J.; Annys, A.; Jannis, D.; Verbeeck, J. |
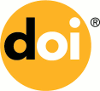
|
|
Title |
Deep learning for automated materials characterisation in core-loss electron energy loss spectroscopy |
Type |
A1 Journal article |
|
Year |
2023 |
Publication |
Scientific reports |
Abbreviated Journal |
|
|
|
Volume |
13 |
Issue |
1 |
Pages |
13724 |
|
|
Keywords |
A1 Journal article; Engineering sciences. Technology; Electron microscopy for materials research (EMAT) |
|
|
Abstract |
Electron energy loss spectroscopy (EELS) is a well established technique in electron microscopy that yields information on the elemental content of a sample in a very direct manner. One of the persisting limitations of EELS is the requirement for manual identification of core-loss edges and their corresponding elements. This can be especially bothersome in spectrum imaging, where a large amount of spectra are recorded when spatially scanning over a sample area. This paper introduces a synthetic dataset with 736,000 labeled EELS spectra, computed from available generalized oscillator strength tables, that represents 107 K, L, M or N core-loss edges and 80 chemical elements. Generic lifetime broadened peaks are used to mimic the fine structure due to band structure effects present in experimental core-loss edges. The proposed dataset is used to train and evaluate a series of neural network architectures, being a multilayer perceptron, a convolutional neural network, a U-Net, a residual neural network, a vision transformer and a compact convolutional transformer. An ensemble of neural networks is used to further increase performance. The ensemble network is used to demonstrate fully automated elemental mapping in a spectrum image, both by directly mapping the predicted elemental content and by using the predicted content as input for a physical model-based mapping. |
|
|
Address |
|
|
|
Corporate Author |
|
Thesis |
|
|
|
Publisher |
|
Place of Publication |
|
Editor |
|
|
|
Language |
|
Wos  |
001052937600046 |
Publication Date |
2023-08-22 |
|
|
Series Editor |
|
Series Title |
|
Abbreviated Series Title |
|
|
|
Series Volume |
|
Series Issue |
|
Edition |
|
|
|
ISSN |
2045-2322 |
ISBN |
|
Additional Links |
UA library record; WoS full record |
|
|
Impact Factor |
4.6 |
Times cited |
|
Open Access |
OpenAccess |
|
|
Notes |
A.A. would like to acknowledge the resources and services used in this work provided by the VSC (Flemish Supercomputer Center), funded by the Research Foundation – Flanders (FWO) and the Flemish Government. J.V. acknowledges the IMPRESS project. The IMPRESS project has received funding from the HORIZON EUROPE framework program for research and innovation under grant agreement n. 101094299. |
Approved |
Most recent IF: 4.6; 2023 IF: 4.259 |
|
|
Call Number |
UA @ admin @ c:irua:198647 |
Serial |
8846 |
|
Permanent link to this record |