|
Records |
Links |
|
Author |
Mary Joy, R.; Pobedinskas, P.; Bourgeois, E.; Chakraborty, T.; Görlitz, J.; Herrmann, D.; Noël, C.; Heupel, J.; Jannis, D.; Gauquelin, N.; D'Haen, J.; Verbeeck, J.; Popov, C.; Houssiau, L.; Becher, C.; Nesládek, M.; Haenen, K. |
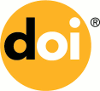
|
|
Title |
Germanium vacancy centre formation in CVD nanocrystalline diamond using a solid dopant source |
Type |
A1 Journal Article |
|
Year |
2023 |
Publication |
Science talks |
Abbreviated Journal |
Science Talks |
|
|
Volume |
5 |
Issue |
|
Pages |
100157 |
|
|
Keywords |
A3 Journal article; Electron microscopy for materials research (EMAT) |
|
|
Abstract |
|
|
|
Address |
|
|
|
Corporate Author |
|
Thesis |
|
|
|
Publisher |
|
Place of Publication |
|
Editor |
|
|
|
Language |
|
Wos |
|
Publication Date |
2023-02-09 |
|
|
Series Editor |
|
Series Title |
|
Abbreviated Series Title |
|
|
|
Series Volume |
|
Series Issue |
|
Edition |
|
|
|
ISSN |
2772-5693 |
ISBN |
|
Additional Links |
UA library record |
|
|
Impact Factor |
|
Times cited |
|
Open Access  |
OpenAccess |
|
|
Notes |
|
Approved |
Most recent IF: NA |
|
|
Call Number |
EMAT @ emat @c:irua:196969 |
Serial |
8791 |
|
Permanent link to this record |
|
|
|
|
Author |
Denisov, N.; Jannis, D.; Orekhov, A.; Müller-Caspary, K.; Verbeeck, J. |


|
|
Title |
Characterization of a Timepix detector for use in SEM acceleration voltage range |
Type |
A1 Journal Article |
|
Year |
2023 |
Publication |
Ultramicroscopy |
Abbreviated Journal |
Ultramicroscopy |
|
|
Volume |
253 |
Issue |
|
Pages |
113777 |
|
|
Keywords |
A1 Journal article; Electron microscopy for materials research (EMAT) |
|
|
Abstract |
Hybrid pixel direct electron detectors are gaining popularity in electron microscopy due to their excellent properties. Some commercial cameras based on this technology are relatively affordable which makes them attractive tools for experimentation especially in combination with an SEM setup. To support this, a detector characterization (Modulation Transfer Function, Detective Quantum Efficiency) of an Advacam Minipix and Advacam Advapix detector in the 15–30 keV range was made. In the current work we present images of Point Spread Function, plots of MTF/DQE curves and values of DQE(0) for these detectors. At low beam currents, the silicon detector layer behaviour should be dominant, which could make these findings transferable to any other available detector based on either Medipix2, Timepix or Timepix3 provided the same detector layer is used. |
|
|
Address |
|
|
|
Corporate Author |
|
Thesis |
|
|
|
Publisher |
|
Place of Publication |
|
Editor |
|
|
|
Language |
|
Wos |
001026912700001 |
Publication Date |
2023-06-08 |
|
|
Series Editor |
|
Series Title |
|
Abbreviated Series Title |
|
|
|
Series Volume |
|
Series Issue |
|
Edition |
|
|
|
ISSN |
0304-3991 |
ISBN |
|
Additional Links |
UA library record; WoS full record |
|
|
Impact Factor |
2.2 |
Times cited |
|
Open Access  |
OpenAccess |
|
|
Notes |
The authors acknowledge the financial support of the Research Foundation Flanders (FWO, Belgium) project SBO S000121N. The authors are grateful to Dr. Lobato for productive discussion of methods. |
Approved |
Most recent IF: 2.2; 2023 IF: 2.843 |
|
|
Call Number |
EMAT @ emat @c:irua:198258 |
Serial |
8815 |
|
Permanent link to this record |
|
|
|
|
Author |
Annys, A.; Jannis, D.; Verbeeck, J.; Annys, A.; Jannis, D.; Verbeeck, J. |
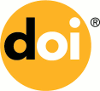
|
|
Title |
Deep learning for automated materials characterisation in core-loss electron energy loss spectroscopy |
Type |
A1 Journal article |
|
Year |
2023 |
Publication |
Scientific reports |
Abbreviated Journal |
|
|
|
Volume |
13 |
Issue |
1 |
Pages |
13724 |
|
|
Keywords |
A1 Journal article; Engineering sciences. Technology; Electron microscopy for materials research (EMAT) |
|
|
Abstract |
Electron energy loss spectroscopy (EELS) is a well established technique in electron microscopy that yields information on the elemental content of a sample in a very direct manner. One of the persisting limitations of EELS is the requirement for manual identification of core-loss edges and their corresponding elements. This can be especially bothersome in spectrum imaging, where a large amount of spectra are recorded when spatially scanning over a sample area. This paper introduces a synthetic dataset with 736,000 labeled EELS spectra, computed from available generalized oscillator strength tables, that represents 107 K, L, M or N core-loss edges and 80 chemical elements. Generic lifetime broadened peaks are used to mimic the fine structure due to band structure effects present in experimental core-loss edges. The proposed dataset is used to train and evaluate a series of neural network architectures, being a multilayer perceptron, a convolutional neural network, a U-Net, a residual neural network, a vision transformer and a compact convolutional transformer. An ensemble of neural networks is used to further increase performance. The ensemble network is used to demonstrate fully automated elemental mapping in a spectrum image, both by directly mapping the predicted elemental content and by using the predicted content as input for a physical model-based mapping. |
|
|
Address |
|
|
|
Corporate Author |
|
Thesis |
|
|
|
Publisher |
|
Place of Publication |
|
Editor |
|
|
|
Language |
|
Wos |
001052937600046 |
Publication Date |
2023-08-22 |
|
|
Series Editor |
|
Series Title |
|
Abbreviated Series Title |
|
|
|
Series Volume |
|
Series Issue |
|
Edition |
|
|
|
ISSN |
2045-2322 |
ISBN |
|
Additional Links |
UA library record; WoS full record |
|
|
Impact Factor |
4.6 |
Times cited |
|
Open Access  |
OpenAccess |
|
|
Notes |
A.A. would like to acknowledge the resources and services used in this work provided by the VSC (Flemish Supercomputer Center), funded by the Research Foundation – Flanders (FWO) and the Flemish Government. J.V. acknowledges the IMPRESS project. The IMPRESS project has received funding from the HORIZON EUROPE framework program for research and innovation under grant agreement n. 101094299. |
Approved |
Most recent IF: 4.6; 2023 IF: 4.259 |
|
|
Call Number |
UA @ admin @ c:irua:198647 |
Serial |
8846 |
|
Permanent link to this record |