|
Record |
Links |
|
Author |
Cai, Y.; Mei, D.; Chen, Y.; Bogaerts, A.; Tu, X. |
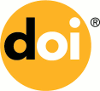
|
|
Title |
Machine learning-driven optimization of plasma-catalytic dry reforming of methane |
Type |
A1 Journal Article |
|
Year  |
2024 |
Publication |
Journal of Energy Chemistry |
Abbreviated Journal |
Journal of Energy Chemistry |
|
|
Volume |
96 |
Issue |
|
Pages |
153-163 |
|
|
Keywords |
A1 Journal Article; Plasma catalysis Machine learning Process optimization Dry reforming of methane Syngas production; Plasma, laser ablation and surface modeling Antwerp (PLASMANT) ; |
|
|
Abstract |
This study investigates the dry reformation of methane (DRM) over Ni/Al2O3 catalysts in a dielectric barrier discharge (DBD) non-thermal plasma reactor. A novel hybrid machine learning (ML) model is developed to optimize the plasma-catalytic DRM reaction with limited experimental data. To address the non-linear and complex nature of the plasma-catalytic DRM process, the hybrid ML model integrates three well-established algorithms: regression trees, support vector regression, and artificial neural networks. A genetic algorithm (GA) is then used to optimize the hyperparameters of each algorithm within the hybrid ML model. The ML model achieved excellent agreement with the experimental data, demonstrating its efficacy in accurately predicting and optimizing the DRM process. The model was subsequently used to investigate the impact of various operating parameters on the plasma-catalytic DRM performance. We found that the optimal discharge power (20 W), CO2/CH4 molar ratio (1.5), and Ni loading (7.8 wt%) resulted in the maximum energy yield at a total flow rate of 51 mL/min. Furthermore, we investigated the relative significance of each operating parameter on the performance of the plasmacatalytic DRM process. The results show that the total flow rate had the greatest influence on the conversion, with a significance exceeding 35% for each output, while the Ni loading had the least impact on the overall reaction performance. This hybrid model demonstrates a remarkable ability to extract valuable insights from limited datasets, enabling the development and optimization of more efficient and selective plasma-catalytic chemical processes. |
|
|
Address |
|
|
|
Corporate Author |
|
Thesis |
|
|
|
Publisher |
|
Place of Publication |
|
Editor |
|
|
|
Language |
|
Wos |
|
Publication Date |
2024-04-25 |
|
|
Series Editor |
|
Series Title |
|
Abbreviated Series Title |
|
|
|
Series Volume |
|
Series Issue |
|
Edition |
|
|
|
ISSN |
2095-4956 |
ISBN |
|
Additional Links |
|
|
|
Impact Factor |
13.1 |
Times cited |
|
Open Access |
|
|
|
Notes |
This project received funding from the European Union’s Hori- zon 2020 research and innovation program under the Marie Sklodowska-Curie grant agreement No. 813393. |
Approved |
Most recent IF: 13.1; 2024 IF: 2.594 |
|
|
Call Number |
PLASMANT @ plasmant @ |
Serial |
9124 |
|
Permanent link to this record |