|
Record |
Links |
|
Author |
McNaughton, B.; Milošević, M.V.; Perali, A.; Pilati, S. |
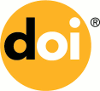
|
|
Title |
Boosting Monte Carlo simulations of spin glasses using autoregressive neural networks |
Type |
A1 Journal article |
|
Year  |
2020 |
Publication |
Physical Review E |
Abbreviated Journal |
Phys Rev E |
|
|
Volume |
101 |
Issue |
5 |
Pages |
053312 |
|
|
Keywords |
A1 Journal article; Condensed Matter Theory (CMT) |
|
|
Abstract |
The autoregressive neural networks are emerging as a powerful computational tool to solve relevant problems in classical and quantum mechanics. One of their appealing functionalities is that, after they have learned a probability distribution from a dataset, they allow exact and efficient sampling of typical system configurations. Here we employ a neural autoregressive distribution estimator (NADE) to boost Markov chain Monte Carlo (MCMC) simulations of a paradigmatic classical model of spin-glass theory, namely, the two-dimensional Edwards-Anderson Hamiltonian. We show that a NADE can be trained to accurately mimic the Boltzmann distribution using unsupervised learning from system configurations generated using standard MCMC algorithms. The trained NADE is then employed as smart proposal distribution for the Metropolis-Hastings algorithm. This allows us to perform efficient MCMC simulations, which provide unbiased results even if the expectation value corresponding to the probability distribution learned by the NADE is not exact. Notably, we implement a sequential tempering procedure, whereby a NADE trained at a higher temperature is iteratively employed as proposal distribution in a MCMC simulation run at a slightly lower temperature. This allows one to efficiently simulate the spin-glass model even in the low-temperature regime, avoiding the divergent correlation times that plague MCMC simulations driven by local-update algorithms. Furthermore, we show that the NADE-driven simulations quickly sample ground-state configurations, paving the way to their future utilization to tackle binary optimization problems. |
|
|
Address |
|
|
|
Corporate Author |
|
Thesis |
|
|
|
Publisher |
|
Place of Publication |
|
Editor |
|
|
|
Language |
|
Wos |
000535862000014 |
Publication Date |
2020-05-28 |
|
|
Series Editor |
|
Series Title |
|
Abbreviated Series Title |
|
|
|
Series Volume |
|
Series Issue |
|
Edition |
|
|
|
ISSN |
1539-3755; 1550-2376 |
ISBN |
|
Additional Links |
UA library record; WoS full record; WoS citing articles |
|
|
Impact Factor |
2.366 |
Times cited |
15 |
Open Access |
|
|
|
Notes |
; The authors thank I. Murray, G. Carleo, and F. RicciTersenghi for useful discussions. Financial support from the FAR2018 project titled “Supervised machine learning for quantum matter and computational docking” of the University of Camerino and from the Italian MIUR under Project No. PRIN2017 CEnTraL 20172H2SC4 is gratefully acknowledged. S.P. also acknowledges the CINECA award under the ISCRA initiative, for the availability of high performance computing resources and support. M.V.M. gratefully acknowledges the Visiting Professorship program at the University of Camerino that facilitated the collaboration in this work. ; |
Approved |
Most recent IF: NA |
|
|
Call Number |
UA @ admin @ c:irua:170244 |
Serial |
6463 |
|
Permanent link to this record |