|
Records |
Links |
|
Author |
Samal, D.; Gauquelin, N.; Takamura, Y.; Lobato, I.; Arenholz, E.; Van Aert, S.; Huijben, M.; Zhong, Z.; Verbeeck, J.; Van Tendeloo, G.; Koster, G. |
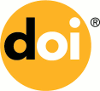
|
|
Title |
Unusual structural rearrangement and superconductivity in infinite layer cuprate superlattices |
Type |
A1 Journal Article |
|
Year |
2023 |
Publication |
Physical review materials |
Abbreviated Journal |
|
|
|
Volume |
7 |
Issue |
5 |
Pages |
054803 |
|
|
Keywords |
A1 Journal article; Electron microscopy for materials research (EMAT) |
|
|
Abstract |
|
|
|
Address |
|
|
|
Corporate Author |
|
Thesis |
|
|
|
Publisher |
|
Place of Publication |
|
Editor |
|
|
|
Language |
|
Wos |
001041792100007 |
Publication Date |
2023-05-30 |
|
|
Series Editor |
|
Series Title |
|
Abbreviated Series Title |
|
|
|
Series Volume |
|
Series Issue |
|
Edition |
|
|
|
ISSN |
2475-9953 |
ISBN |
|
Additional Links |
UA library record; WoS full record |
|
|
Impact Factor |
3.4 |
Times cited |
|
Open Access  |
OpenAccess |
|
|
Notes |
Air Force Office of Scientific Research; European Office of Aerospace Research and Development, FA8655-10-1-3077 ; Office of Science, DE-AC02-05CH11231 ; National Science Foundation, DMR-1745450 ; Seventh Framework Programme, 278510 ; Bijzonder Onderzoeksfonds UGent; |
Approved |
Most recent IF: 3.4; 2023 IF: NA |
|
|
Call Number |
EMAT @ emat @c:irua:196973 |
Serial |
8790 |
|
Permanent link to this record |
|
|
|
|
Author |
Lobato, I.; De Backer, A.; Van Aert, S. |


|
|
Title |
Real-time simulations of ADF STEM probe position-integrated scattering cross-sections for single element fcc crystals in zone axis orientation using a densely connected neural network |
Type |
A1 Journal Article |
|
Year |
2023 |
Publication |
Ultramicroscopy |
Abbreviated Journal |
Ultramicroscopy |
|
|
Volume |
251 |
Issue |
|
Pages |
113769 |
|
|
Keywords |
A1 Journal article; Electron microscopy for materials research (EMAT) |
|
|
Abstract |
Quantification of annular dark field (ADF) scanning transmission electron microscopy (STEM) images in terms
of composition or thickness often relies on probe-position integrated scattering cross sections (PPISCS). In
order to compare experimental PPISCS with theoretically predicted ones, expensive simulations are needed for
a given specimen, zone axis orientation, and a variety of microscope settings. The computation time of such
simulations can be in the order of hours using a single GPU card. ADF STEM simulations can be efficiently
parallelized using multiple GPUs, as the calculation of each pixel is independent of other pixels. However, most
research groups do not have the necessary hardware, and, in the best-case scenario, the simulation time will
only be reduced proportionally to the number of GPUs used. In this manuscript, we use a learning approach and
present a densely connected neural network that is able to perform real-time ADF STEM PPISCS predictions as
a function of atomic column thickness for most common face-centered cubic (fcc) crystals (i.e., Al, Cu, Pd, Ag,
Pt, Au and Pb) along [100] and [111] zone axis orientations, root-mean-square displacements, and microscope
parameters. The proposed architecture is parameter efficient and yields accurate predictions for the PPISCS
values for a wide range of input parameters that are commonly used for aberration-corrected transmission
electron microscopes. |
|
|
Address |
|
|
|
Corporate Author |
|
Thesis |
|
|
|
Publisher |
|
Place of Publication |
|
Editor |
|
|
|
Language |
|
Wos |
001011617200001 |
Publication Date |
2023-06-01 |
|
|
Series Editor |
|
Series Title |
|
Abbreviated Series Title |
|
|
|
Series Volume |
|
Series Issue |
|
Edition |
|
|
|
ISSN |
0304-3991 |
ISBN |
|
Additional Links |
UA library record; WoS full record |
|
|
Impact Factor |
2.2 |
Times cited |
|
Open Access  |
Open_Access |
|
|
Notes |
This work was supported by the European Research Council (Grant 770887 PICOMETRICS to S. Van Aert). The authors acknowledge financial support from the Research Foundation Flanders (FWO, Belgium) through project fundings (G034621N and G0A7723N) and a postdoctoral grant to A. De Backer. S. Van Aert acknowledges funding from the University of Antwerp Research fund (BOF), Belgium. |
Approved |
Most recent IF: 2.2; 2023 IF: 2.843 |
|
|
Call Number |
EMAT @ emat @c:irua:197275 |
Serial |
8812 |
|
Permanent link to this record |