|
Records |
Links |
|
Author |
Chai, Z.-N.; Wang, X.-C.; Yusupov, M.; Zhang, Y.-T. |
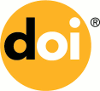
|
|
Title  |
Unveiling the interaction mechanisms of cold atmospheric plasma and amino acids by machine learning |
Type |
A1 Journal article |
|
Year |
2024 |
Publication |
Plasma processes and polymers |
Abbreviated Journal |
|
|
|
Volume |
|
Issue |
|
Pages |
1-26 |
|
|
Keywords |
A1 Journal article; Plasma Lab for Applications in Sustainability and Medicine – Antwerp (PLASMANT) |
|
|
Abstract |
Plasma medicine has attracted tremendous interest in a variety of medical conditions, ranging from wound healing to antimicrobial applications, even in cancer treatment, through the interactions of cold atmospheric plasma (CAP) and various biological tissues directly or indirectly. The underlying mechanisms of CAP treatment are still poorly understood although the oxidative effects of CAP with amino acids, peptides, and proteins have been explored experimentally. In this study, machine learning (ML) technology is introduced to efficiently unveil the interaction mechanisms of amino acids and reactive oxygen species (ROS) in seconds based on the data obtained from the reactive molecular dynamics (MD) simulations, which are performed to probe the interaction of five types of amino acids with various ROS on the timescale of hundreds of picoseconds but with the huge computational load of several days. The oxidative reactions typically start with H-abstraction, and the details of the breaking and formation of chemical bonds are revealed; the modification types, such as nitrosylation, hydroxylation, and carbonylation, can be observed. The dose effects of ROS are also investigated by varying the number of ROS in the simulation box, indicating agreement with the experimental observation. To overcome the limits of timescales and the size of molecular systems in reactive MD simulations, a deep neural network (DNN) with five hidden layers is constructed according to the reaction data and employed to predict the type of oxidative modification and the probability of occurrence only in seconds as the dose of ROS varies. The well-trained DNN can effectively and accurately predict the oxidative processes and productions, which greatly improves the computational efficiency by almost ten orders of magnitude compared with the reactive MD simulation. This study shows the great potential of ML technology to efficiently unveil the underpinning mechanisms in plasma medicine based on the data from reactive MD simulations or experimental measurements. In this study, since reactive molecular dynamics simulation can currently only describe interactions between a few hundred atoms in a few hundred picoseconds, deep neural networks (DNN) are introduced to enhance the simulation results by predicting more data efficiently. image |
|
|
Address |
|
|
|
Corporate Author |
|
Thesis |
|
|
|
Publisher |
|
Place of Publication |
|
Editor |
|
|
|
Language |
|
Wos |
001202061200001 |
Publication Date |
2024-04-15 |
|
|
Series Editor |
|
Series Title |
|
Abbreviated Series Title |
|
|
|
Series Volume |
|
Series Issue |
|
Edition |
|
|
|
ISSN |
1612-8850 |
ISBN |
|
Additional Links |
UA library record; WoS full record |
|
|
Impact Factor |
3.5 |
Times cited |
|
Open Access |
|
|
|
Notes |
|
Approved |
Most recent IF: 3.5; 2024 IF: 2.846 |
|
|
Call Number |
UA @ admin @ c:irua:205512 |
Serial |
9181 |
|
Permanent link to this record |
|
|
|
|
Author |
Vermang, B.; Brammertz, G.; Meuris, M.; Schnabel, T.; Ahlswede, E.; Choubrac, L.; Harel, S.; Cardinaud, C.; Arzel, L.; Barreau, N.; van Deelen, J.; Bolt, P.-J.; Bras, P.; Ren, Y.; Jaremalm, E.; Khelifi, S.; Yang, S.; Lauwaert, J.; Batuk, M.; Hadermann, J.; Kozina, X.; Handick, E.; Hartmann, C.; Gerlach, D.; Matsuda, A.; Ueda, S.; Chikyow, T.; Felix, R.; Zhang, Y.; Wilks, R.G.; Baer, M. |
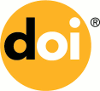
|
|
Title  |
Wide band gap kesterite absorbers for thin film solar cells: potential and challenges for their deployment in tandem devices |
Type |
A1 Journal article |
|
Year |
2019 |
Publication |
Sustainable Energy & Fuels |
Abbreviated Journal |
|
|
|
Volume |
3 |
Issue |
9 |
Pages |
2246-2259 |
|
|
Keywords |
A1 Journal article; Engineering sciences. Technology; Electron microscopy for materials research (EMAT) |
|
|
Abstract |
This work reports on developments in the field of wide band gap Cu2ZnXY4 (with X = Sn, Si or Ge, and Y = S, Se) kesterite thin film solar cells. An overview on recent developments and the current understanding of wide band gap kesterite absorber layers, alternative buffer layers, and suitable transparent back contacts is presented. Cu2ZnGe(S,Se)(4) absorbers with absorber band gaps up to 1.7 eV have been successfully developed and integrated into solar cells. Combining a CdS buffer layer prepared by an optimized chemical bath deposition process with a 1.36 eV band gap absorber resulted in a record Cu2ZnGeSe4 cell efficiency of 7.6%, while the highest open-circuit voltage of 730 mV could be obtained for a 1.54 eV band gap absorber and a Zn(O,S) buffer layer. Employing InZnOx or TiO2 protective top layers on SnO2:In transparent back contacts yields 85-90% of the solar cell performance of reference cells (with Mo back contact). These advances show the potential as well as the challenges of wide band gap kesterites for future applications in high-efficiency and low-cost tandem photovoltaic devices. |
|
|
Address |
|
|
|
Corporate Author |
|
Thesis |
|
|
|
Publisher |
|
Place of Publication |
|
Editor |
|
|
|
Language |
|
Wos |
000482057500004 |
Publication Date |
2019-06-14 |
|
|
Series Editor |
|
Series Title |
|
Abbreviated Series Title |
|
|
|
Series Volume |
|
Series Issue |
|
Edition |
|
|
|
ISSN |
|
ISBN |
|
Additional Links |
UA library record; WoS full record; WoS citing articles |
|
|
Impact Factor |
|
Times cited |
2 |
Open Access |
|
|
|
Notes |
; This project has received funding from the European Union's Horizon 2020 Research and Innovation Program under grant agreement No. 640868. The synchrotron radiation experiments were performed at the SPring-8 beamline BL15XU with the approval of the NIMS Synchrotron X-ray Station (Proposals 2016A4600, 2016B4601, and 2017A4600) and at BESSY II with the approval of HZB. B. Vermang has received funding from the European Research Council (ERC) under the European Union's Horizon 2020 Research and Innovation Programme (grant agreement no. 715027). ; |
Approved |
Most recent IF: NA |
|
|
Call Number |
UA @ admin @ c:irua:161785 |
Serial |
5404 |
|
Permanent link to this record |